Today on the Salesforce Admins Podcast, we talk to Joy Shutters-Helbing, Senior Manager of Salesforce Practice at Captech and Salesforce MVP Hall-of-Famer, and Mike Reynolds, Senior Product Marketing Manager at Slack. Join us as we chat about creating community content, navigating conference submissions, and their new podcast, The JAM. You should subscribe for the full […]
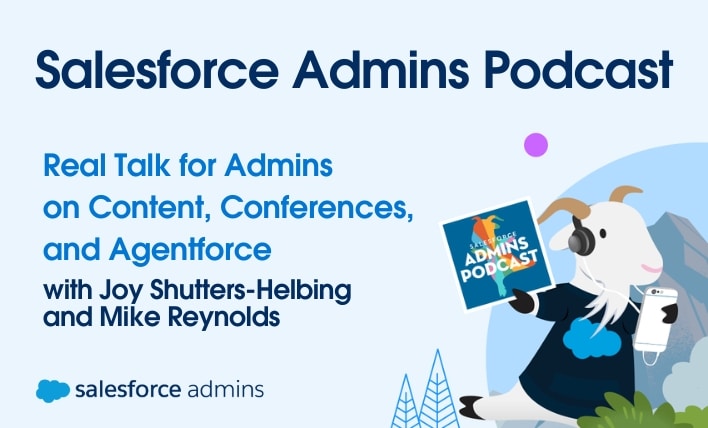