Today on the Salesforce Admins Podcast, we talk to Andrew Russo, Salesforce Architect at BACA Systems. Join us as we chat about Salesforce Foundations and why it’s a game changer for solo admins and small orgs. You should subscribe for the full episode, but here are a few takeaways from our conversation with Andrew Russo. […]
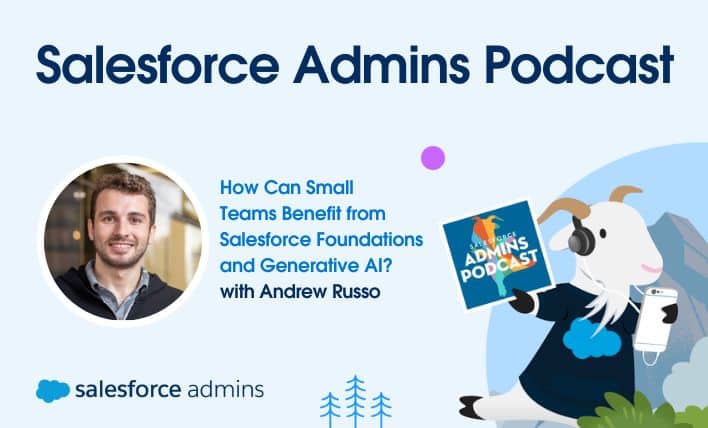