Chicken, meet egg As admins, we’re often confronted with a classic conundrum: How do you convince an organization to adopt new technology without having the technology to demonstrate it in the first place? This is compounded when the technology you want to demonstrate requires other technologies you may not have access to show. For example, […]
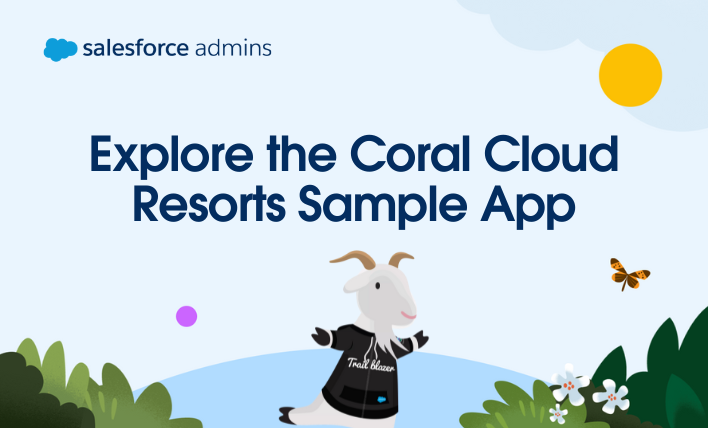