You know artificial intelligence (AI) is officially everywhere when your 73-year-young mother asks you if AI can create a birthday card design for one of her friends. Which is another way of saying, it’s most likely crept into your business conversations with stakeholders and users. If not, then let’s be proactive Salesforce Admins and get […]
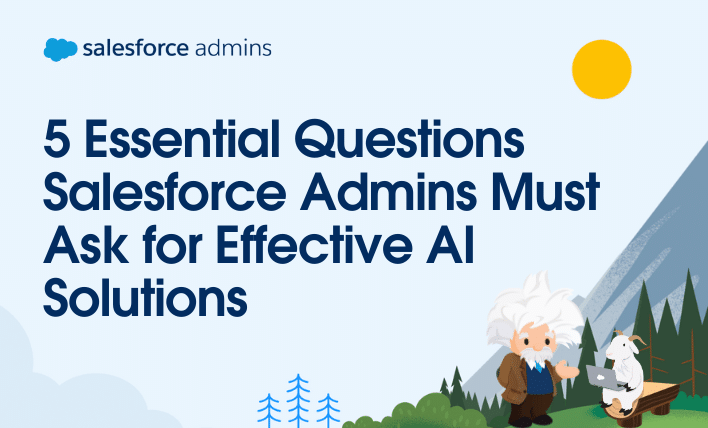